Note:
This topic has been translated from a Chinese forum by GPT and might contain errors.Original topic: TiDB快速起步学习笔记一
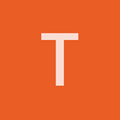
[
History of Distributed Systems
](#%E5%88%86%E5%B8%83%E5%BC%8F%E7%B3%BB%E7%BB%9F%E7%9A%84%E5%8E%86%E5%8F%B2)
In 2006, Google introduced the three pillars of big data:
* GFS -- Solved the distributed file system problem
* Google BigTable - Solved the distributed key-value problem
* Google MapReduce - Solved how to perform distributed computing and analysis on distributed file systems and distributed KV storage.
Main challenges of distributed computing
* How to maximize the implementation of divide and conquer
* How to achieve global consistency
* How to perform fault tolerance and partial failure tolerance
* How to deal with unreliable networks and network partitions
Famous CAP theorem in distributed systems
- Consistency - Replica consistency
- Availability
- Partition Tolerance
- CA, CP, AP
Transactions: ACID
Two different consistencies: the former describes replica consistency, the latter describes transaction consistency.
RPO: Recovery Point Objective, mainly refers to the amount of data loss that the business system can tolerate.
RTO: Recovery Time Objective, mainly refers to the maximum time that the business can tolerate being out of service.
[
TiDB Highly Layered Architecture
](TiDB%20%E9%AB%98%E5%BA%A6%E5%88%86%E5%B1%82%E6%9E%B6%E6%9E%84)
Elasticity is the core consideration of the entire architecture design. TiDB is mainly divided into three layers logically:
* Technical engine supporting standard SQL - TIDB Server
* Distributed storage engine - TiKV
- Metadata management and scheduling engine - Placement Driver (PD)
- Cluster metadata management, including shard distribution, topology, etc.
- Distributed transaction ID allocation
- Scheduling center
Core of the database: Data structure
TiKV single node chose the RocksDB engine based on LSM-tree:
- RocksDB is a very mature LSM-tree storage engine
- Supports atomic batch write
- Lock-free snapshot read (Snapshot)
- This feature plays a role in data replica migration.
[
TiKV
](tikv)
TiKV system adopts range data sharding algorithm.
Distributed transaction model
- Decentralized two-phase commit
- Global timestamping through PD (TSO)
- –4M timestamps per second
- Each TiKV node allocates a separate area to store lock information (CF Lock)
- Google Percolator transaction model
- TiKV supports complete transaction KV API
- Default optimistic transaction model
- Also supports pessimistic transaction model (version 3.0+)
- Default isolation level: Snapshot Isolation
SQL relational model
Implementing logical tables on KV:
HTAP
Using Spark to alleviate the computational power problem of the data middle platform: can only provide low-concurrency heavyweight queries.
Physical isolation is the best resource isolation
Column storage is naturally friendly to OLAP queries - Tiflash
Row-column data synchronization - Raft-based best solution
MPP engine - parallel computing
The TiDB database has already implemented the following features in HTAP technology:
- Columnar storage has achieved real-time write capability
- MPP solves node scalability and parallel computing
- Enables Spark to run on TiKV.