Note:
This topic has been translated from a Chinese forum by GPT and might contain errors.Original topic: 【TiDBer 唠嗑茶话会 115】TiDB 支持向量功能,你最想拿它做什么?
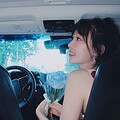
This week, I saw many friends sharing @hey-hoho’s article in their Moments
Everyone is very excited about TiDB’s vector support feature.
Currently, TiDB serverless already supports vector functionality!
Trial Entry
Waitlist application entry: https://tidb.cloud/ai
Experience entry: https://tidbcloud.com
This Topic:
What do you most want to do with TiDB’s vector support feature?
Do you have a need for TiDB’s vector support?
In what scenarios would you use this feature?
What are your thoughts or suggestions on the future launch of TiDB’s vector functionality?
Interpretation of vector from @Icemap on the 57th floor:
I’ll start the discussion here. We are currently using the Vector feature in a specific RAG scenario.
RAG stands for retrieval-augmented generation. This is the type of Apps we refer to as retrieval-augmented-generation, which is the most common method of constructing Apps in the current AI field and is widely used.
For example, if you have used ChatGPT, you will be impressed by its ability to speak nonsense seriously. This is called the “hallucination” of LLM, a situation where it loses attention.
So how can we simply solve this problem? The answer is RAG. We can first retrieve some reliable materials for the question, provide LLM with some reliable context as augmentation, and then let it generate new content. This can significantly improve the accuracy of the answers.
Here is a more specific example. We are working on tidb.ai, hoping to use large models and our own documentation to answer questions about TiDB. The steps can be as follows:
- The user asks a question about TiDB
- Search for relevant TiDB documents based on the question
- Use the documents to fill in the LLM’s Prompt
- Let the LLM generate the output in the required format
Here comes a problem, how to search for relevant TiDB documents based on the question? This brings up another AI feature called Embedding. It generates a feature vector for a piece of text, and we can compare the Embedding Vector distances of two pieces of text. The closer the distance, the more relevant the two pieces of text are. Therefore, we can optimize the above process as follows:
- Pre-embed TiDB documents, store the text and its corresponding Vector in TiDB
- The user asks a question about TiDB, performs Embedding, and uses the vector distance calculation in TiDB to search for the most relevant documents in TiDB
- Use the documents to fill in the LLM’s Prompt
- Let the LLM generate the output in the required format
This is one of the reasons why we need the Vector feature. Additionally, how to generate Embedding and how to Retrieve are optimization points. This is also the part we are working on. However, the Vector feature of TiDB is indispensable here. Here is a comparison chart if we use an external Vector database versus TiDB’s built-in Vector.
Of course, this is just one use case of Vector. We look forward to more usage methods from everyone.
Participation Rewards:
Participate in the discussion and get 30 points & experience!
Event Time:
2024.4.19 - 2024.4.25